Galaxies To Eyes: New Deepfake Detection Method
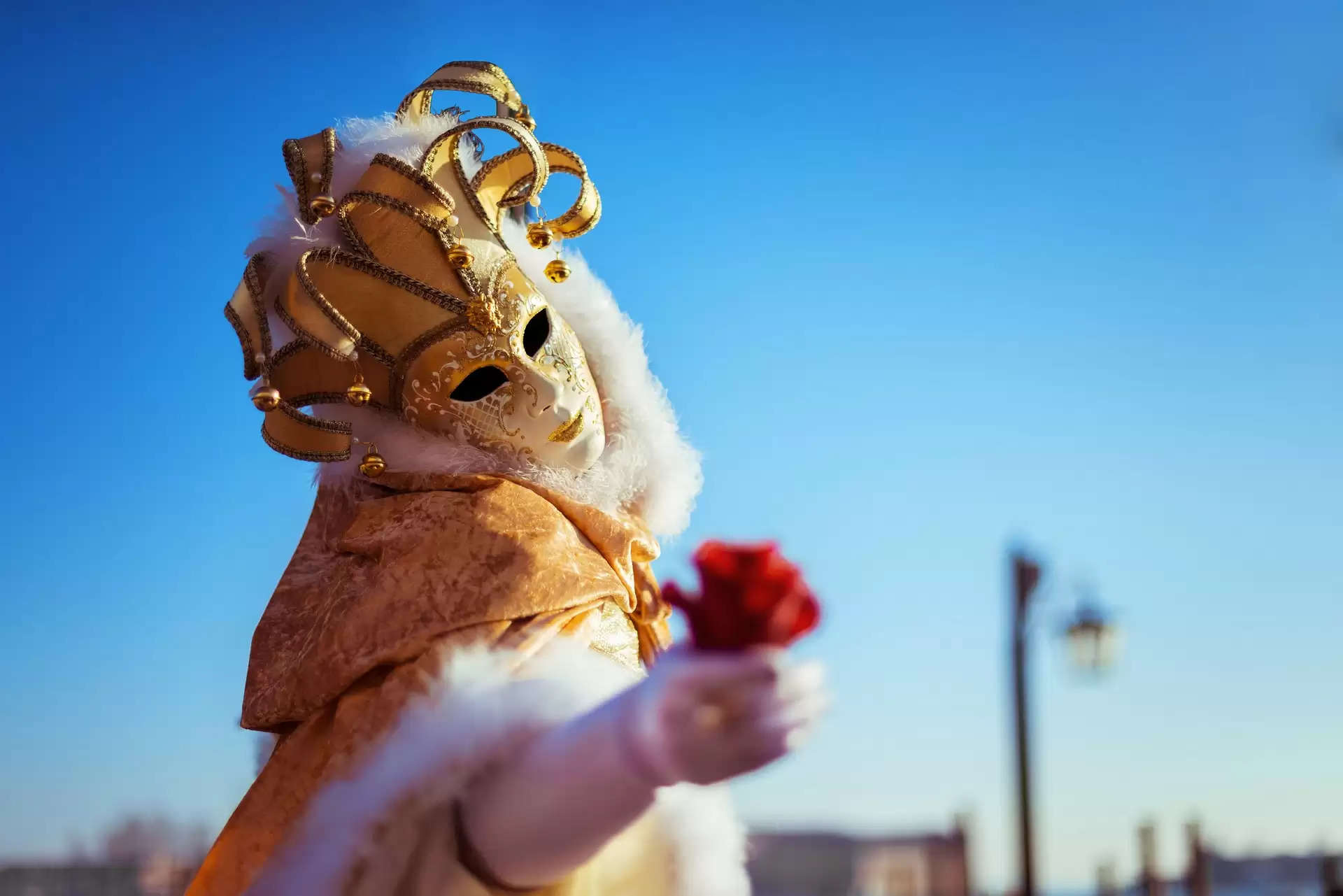
In today's world, the creation of artificial intelligence (AI) images is accessible to the general public, making the ability to detect fake pictures, particularly deepfakes of individuals, increasingly crucial.
Recent research reveals a compelling method to identify these AI-generated fakes by analyzing human eyes, akin to how astronomers study images of galaxies.
The Key to Detection: Eye Reflections
The core of this groundbreaking research lies in the reflections observed in a person's eyeballs. If these reflections are consistent, the image is likely of a real human.
In contrast, inconsistencies suggest the image might be a deepfake. The reflections in a real person's eyes align with physical laws, whereas fake ones do not.
Methodology: Borrowing from Astronomy
Researchers employed techniques from astronomy to analyze light reflections on the eyeballs of people in both real and AI-generated images.
They assessed the consistency between reflections in the left and right eyes. Real images typically exhibit similar reflections in both eyes, while fake images often do not.
The Role of Morphological Features
The research team utilized automated methods to detect reflections and analyze their morphological features using the CAS (concentration, asymmetry, smoothness) and Gini indices.
These indices helped compare the similarity between the left and right eyeball reflections. Differences in these reflections often indicated the presence of deepfakes.
Gini Coefficient: A Measure of Light Distribution
The Gini coefficient, traditionally used to measure light distribution in images of galaxies, played a significant role in this research. It measures how light is spread across an image’s pixels.
A Gini value of 0 indicates even light distribution, while a value of 1 signifies all light concentrated in a single pixel. This method helped differentiate between real and fake images based on light distribution patterns in the eyes.
Limitations and Future Prospects
While the CAS parameters were not as effective in predicting fake eyes, the research underscores that this method is not a foolproof solution for detecting fake images. There are instances of false positives and false negatives, highlighting that this approach is not all-encompassing.
However, it provides a foundational strategy in the ongoing battle to identify deepfakes.
A New Tool in the Fight Against Deepfakes
This innovative research marks a significant step in the fight against the proliferation of AI-generated deepfakes. By leveraging techniques from astronomy and focusing on eye reflections, researchers have developed a novel method to identify fake images.
Although not infallible, this approach offers a promising basis for future advancements in deepfake detection, providing a new tool in the ongoing efforts to maintain the integrity of visual media.